The COVID-19 pandemic has severely affected human life across the globe. Tackling the spread requires risk assessment at an individual level. In this post, we propose a risk model to quantify the risk of infection and vulnerability for COVID-19 using individual-level demographic and behavioral data. The risk model that we develop can help government agencies develop a situational awareness system to tackle the spread of COVID-19. Using our risk model, we can assign risk scores to every individual in the population, which can then help government agencies make effective decisions to contain the disease spread. For example, older people, who are vulnerable to COVID-19, will be assigned high-risk scores, and in turn, will be notified to maintain strict social distancing measures. Overall, our risk modeling approach quantifies the COVID-19 risk both at an individual as well as on a geographical level.
Modeling Approach
The features that we consider in our risk model come from individual behavior and demographics. We first compute normalized risk factors for each attribute and then combine them using a weighted sum model with configurable weights to provide a risk score ranging between 0 and 1. For calculating the risk factors, we rely on publicly available data and insights obtained from published research. The figure below summarizes our approach and highlights the basis for each of those features. Where the data is not available, we use a sigmoid function to get the risk factor assuming that a monotonic relationship exists between the feature value and its risk factor.
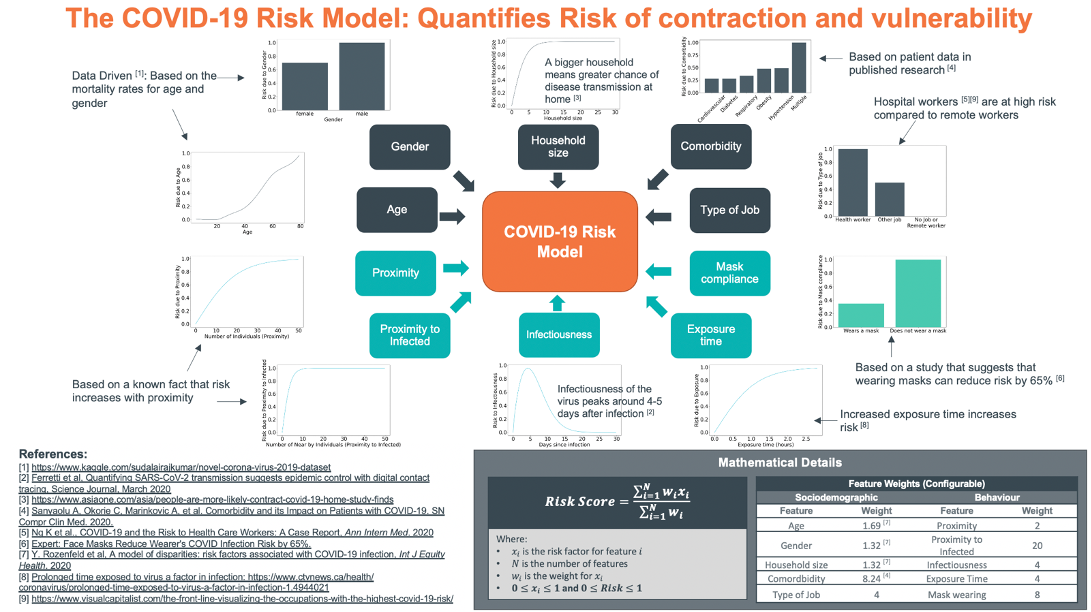
We give a more detailed description of the features in our model below:
- Age and Gender. Mortality data for COVID-19 shows that the CFR is higher in older age people and the male gender. We use the interpolated CFR values as the risk factors for these two features.
- Household size. A study has shown that people are more likely to get infected by their household members. Therefore, we assume that the risk increases with household size.
- Type of Job. A detailed report highlights that healthcare workers are at high risk of contracting COVID-19, thus making it a significant factor to consider in the risk model.
- Comorbidity. A wide range of studies has assessed the impact of different comorbidities on patients having COVID-19. As per a published study, the comorbidities having the highest impact on the COVID-19 patients, as observed from the confirmed hospitalization data, were hypertension, obesity, cardiovascular diseases, respiratory diseases, and diabetes. We consider these comorbidities in our work and use the odds-ratios obtained from such studies to compute the corresponding risk factor.
- Proximity and Proximity to infected. The primary mode of transmission of COVID-19 is through close contact with infected individuals. So, the risk of infection for any individual is highly dependent upon the number of individuals nearby in the same location at a given time (proximity). Furthermore, the risk will increase further if there are infected people present as well (proximity to infected). We assume that the risk of infection will increase if an individual’s proximity to others is high
- Infectiousness. Published research shows that the likelihood of virus transmission varies as a function of the days since infection. We use this model to get the corresponding risk factor.
- Exposure time. The time spent near other individuals quantifies the exposure time. As the exposure increases, the risk of infection increases as well.
- Mask-Wearing. Finally, we consider mask-wearing since a study suggests that mask-wearing can reduce the risk of infection by up to 65%.
In a previous post, we presented a
simulation framework that allows us to simulate the spread of COVID-19 in urban environments. We use the framework here to demonstrate the proposed risk model by using the simulated data to compute the risk scores for every individual in the simulations. The individual risk scores are then aggregated on a location level to get geographical risk scores as well. For visualization, we build dashboards for both the individual as well as the geographical risk scoring.
Geographical Risk Scoring Dashboard
In this dashboard, we can perform a visual analysis of the risk of different locations in a given geographical region, and in turn identify hotspots. In a realistic scenario, such analysis allows the governing bodies to take efficient decisions for the controlling the spread of the disease. A snapshot of the dashboard is shown below.
Individual Risk Comparison Dashboard
This dashboard allows us to visualize the individual risk scores for comparative analysis. A snapshot of the dashboard is given below for two sample individuals in the simulated data. In the example, on the left, we have an individual with a low vulnerability score, while on the right, we have another individual with a high vulnerability score. However, we see that only the individual on the left gets infected. The reason is that the
risk of infection is primarily dependent upon the behavior. The individual on the left has high proximity to others, which means that the chance of getting infected is more. Eventually, we see that the individual indeed gets infected. On the other hand, the individual on the right does not travel a lot, and her proximity to others remains low, and this prevents her from getting infected.
.png)
In conclusion, in this post, we presented a generic risk model for COVID-19 that considers several individual features to quantify the COVID-19 risk and can help government agencies develop efficient situational awareness systems for monitoring and controlling the spread of COVID-19.
Acknowledgement. The work is part of Teradata’s COVID360 initiative led by Christopher Jackson, where the aim is to help countries restart their economies in the post-COVID world.
(Author):
Christopher Jackson
Chris has more than 30 years of leadership experience in technology sales and delivery. His expertise includes advanced analytics, data science, artificial intelligence, enterprise data warehousing, systems integration, strategic planning, business process automation, collaboration, and project/program management. He also has experience in financial services/banking, government, telecommunications, and healthcare industries. At Teradata, Chris leads the teams of Pre-Sales Solution Engineers at Teradata South Asia & Korea. He is currently leading the COVID360 initiative where the goal is to develop a COVID 360 Situational Awareness and Actionable Intelligence Framework that can be used by nations to manage the outbreak and post-COVID-19 recovery.
View all posts by Christopher Jackson
(Author):
Muhammad Jawad Khokhar
Jawad is an experienced Data Scientist with a PhD in Computer Science from Inria Sophia Antipolis, France. He has extensive experience in the Telecom sector where he has undertaken several projects in network operations, modeling, analysis, and optimization. At Teradata, he has worked on Data Science projects for several industries including Telecom, Healthcare, and Oil & Gas. Overall, his expertise lies in data science, machine learning, and Internet measurements. Currently, he is part of Teradata’s COVID360 initiative where he has developed a framework for modeling, simulation, and risk profiling of COVID-19.
View all posts by Muhammad Jawad Khokhar