What if your computer knew what you were typing? What if it knew you were bullying someone online? Would you still type those words, aware that your computer knows you are being hurtful?
Artificial intelligence is already being used around the world online. Gmail now uses an AI-based programme to suggest responses to emails. The iPhone has Siri, which listens to your commands and does her — or his, if you alter the default settings — best to provide answers. Even Grammarly, an internet-browser adapter, reads what you write and suggests edits to improve your correspondence. But do any of these methods actually understand your writing?
True AI should be able to understand the context of what you are writing, determine the overarching purpose of the communication, and perhaps even the reason behind the writing it in the first place. From there, it could determine if what you’re saying is positive, neutral, or negative. If it deems you to be writing something meant to be hurtful towards someone else, it could seize control of your keyboard, thus helping end online bullying or preventing you from sending that angry email immediately after a heated conversation.
Understand me
Before we can end online bullying, we need to determine how to make computers understand what we are actually typing. For this, we look to word definitions.
If an AI can understand the meaning of the words you use, as well as the context within which those words are being used, it could differentiate between the 28 percent of words that have multiple meanings, thus, understanding what you’re talking about.
Within text analytics, this is done by pulling apart sentences, then putting them back together after determining their meanings.
Each sentence is split into individual words. Stop words (e.g., it, the, a) are removed, and each word is matched to every possible synset — or sets of synonyms — and definition. Words are also matched based on type, so if a word should be a noun, we can match it only with possible synsets and definitions that are also nouns. Joining by word type can help shrink the number of possibilities, reducing the computational time of the whole process.
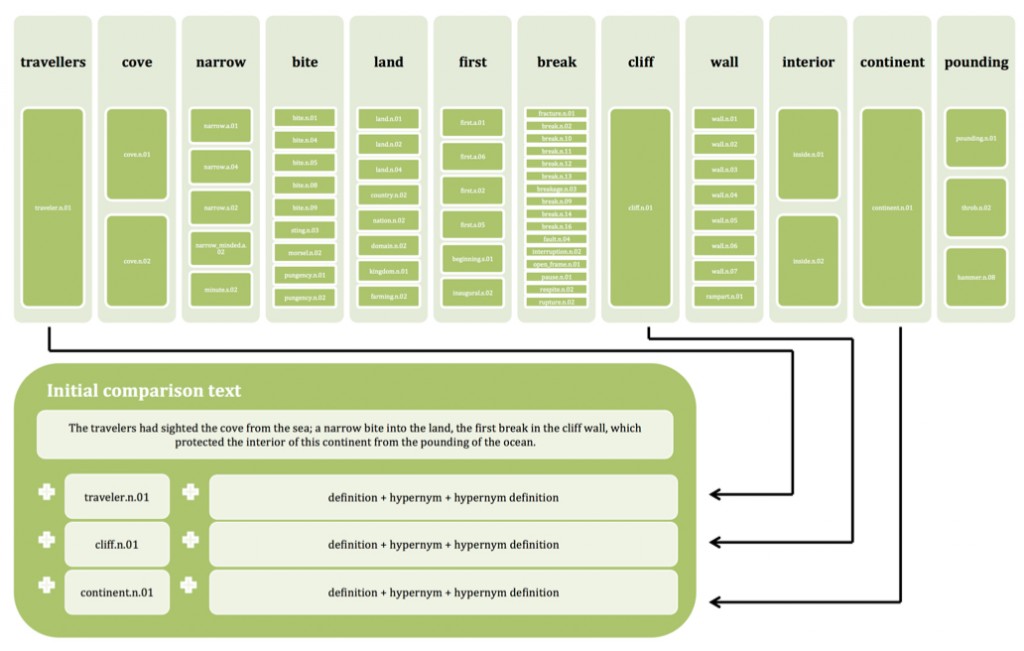
Figure 1: Each word in the sentence is matched to possible definitions in the dictionary. Not all words have definitions in the dictionary based on the exact version of a word.
Cosine distances
The most likely word definitions are determined by minimising the cosine distance between all words in a sentence. Cosine distances are calculated between two sets of tokens, and tokens are derived from two strings.
Still following? Good.
The initial set of tokens comprises of the words from the original sentence, plus the synsets, definitions, hypernyms (the next most general version of that word), and hypernym definitions of all words that only have a single possibility. The second set of tokens includes the words from the next possible word, definition, hypernym, and hypernym definition combination.
Figure 2: The initial comparison text is compared to each possible definition of a word. The definition that results in the lowest cosine distance is selected to be the most likely and is added to the initial comparison text.
The greater the similarity between two sets of words, the smaller the cosine distance between them. The word and definition combination that obtains the smallest cosine distance is joined to the initial set of words. Then the analysis is repeated with the next word possibility.
Figure 3: Once one word has been decided on, it is added with its definition to the initial comparison text and compared to the possible definitions of the next word.
The process continues until all words have been assigned. In order to account for word definitions that have equal cosine distances, this process is repeated around 1,000 times, changing the order in which the definitions are compared. This way, the resulting frequency of those definitions will even out and reject anything with no statistical significance.
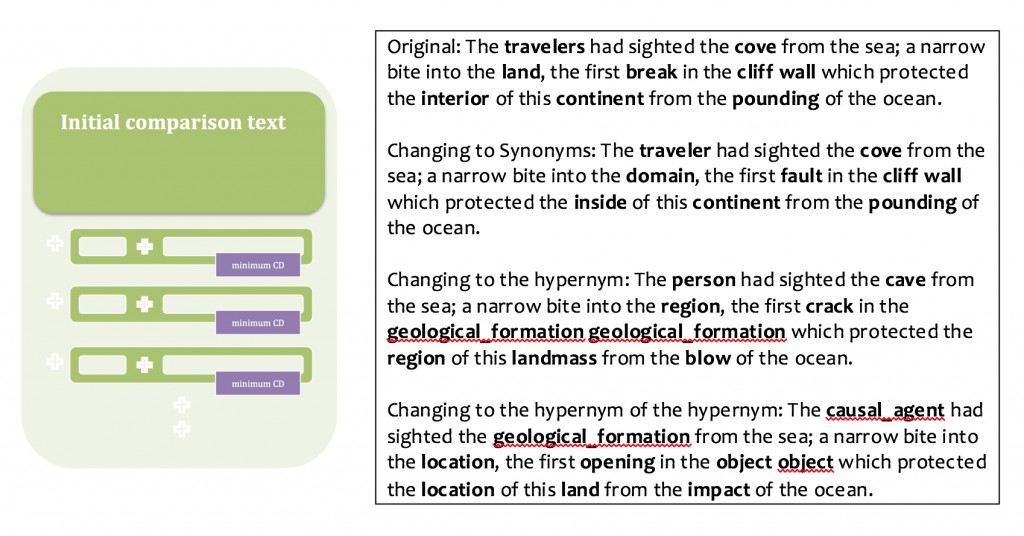
Figure 4: The final set of words/synsets.
Confidence intervals
The test for statistical significance determines if the most frequent word definition selected is statistically greater than the second most frequent word definition. Confidence intervals (CI) of both frequencies are also calculated to see any overlap.

Figure 5: If the lower bound of the CI for the top word does not intersect the upper bound of the second top word, the top word is statistically significant.
This type of analysis is not perfect. The description of each word definition in the dictionary plays a large part in making the connections to the right words. If the dictionary doesn’t have enough information within its definitions, then it can be difficult to get the right answer. By increasing the knowledge of the AI dictionary to include, perhaps, example sentences of words being used in context, this may increase the reliability of selecting the correct word definition in each case. But there is still more work to be done here.
Analysing the future
Online bullying is a major problem facing young people today. Bullying was bad enough in the schoolyard, but at least you could run away or attempt to ignore it. The penetration of connected technology and the pervasiveness of social presence in today’s society means that today there is almost no escape.
A safer world could include the eradication of hateful speech online — hopefully with a smart enough contextual analyser to differentiate between bullying and stating a strong opinion. This method may not work at present, but imagine a parental blocker that can analyse the text being written online and determine if it was positive or negative. What if that technology could stop hateful messages from even being seen? If you were sent something harmful, it could be flagged or censored, avoiding the tragedy of being bullied online.
If AI could achieve this, we could use it to take away the power of online bullies … or at least email their parents!
As a relatively new addition (since March 2017) to the Teradata team, Elise Hampton works as a data scientist within Think Big Analytics. At the Research School of Astronomy & Astrophysics in Australia, Elise first encountered machine learning, which has driven her to shift careers from astronomical research to data science. Elise’s interests (in data science) include finding ways of using machine learning to 'do the hard work for us’, using computers to understand language and people, and solving the world’s problems. Outside of work you may find Elise seeking out famous people at Sci-fi conventions, pondering whether lightsabers will ever be possible, and cosplaying as Disney Princesses or pop-culture heroes around Australia.
View all posts by Elise Hampton