On February 5, the number of confirmed COVID-19 cases jumped 45% when authorities included clinically diagnosed patients in Hubei province in China
[1]. The reason for this uptick was largely publicized as a modification to national treatment guidelines, adding 13,332 cases to the confirmed list. In other words, until the use of more specific diagnostics through nucleic acid testing kits and CT scans, there was a large number of
unconfirmed cases (patients that present but are not diagnosed) of the infection in Hubei, as their health systems were representing a large percentage of presumed positive cases as “Pneumonia” and “Influenza” instead of viral pneumonia caused by COVID-19. It exemplifies how little confidence there is in relying on ‘reported’ or ‘discovered’ cases early in epidemics.
This leads us to today, where the suspected number of reported cases in the United States is 4138 as of Monday, March 13
th.
[2] However, two glaring system challenges are the lack of approved tests to confirm COVID-19 infections, as CT scans are not being endorsed by the American College of Radiology as a diagnostic mechanism
[3], and only approximately 41,000 (3/13 numbers) individuals have been tested
[4]. Second, there are an even larger number of
undocumented cases (individuals that provide no trace of data for health systems or public health agencies to track). An epidemiology simulation of the Wuhan data, indicates a staggering 86% of cases in the population are undocumented.
[5] We’re going to tackle the former in this discussion.
At the start of this crisis, we were able to use travel history as a key attribute in differentiating Influenza from COVID-19, focusing on travel to countries with high incidence rates in early February (China, South Korea, Iran) and therefore weight this factor into both presumed diagnoses or, as data scientists, as unconfirmed cases. Additionally, the epidemiology community notes the vast majority of cases go undocumented for a variety of reasons (patient doesn’t present to the hospital, patient not tested or individual refuses testing, and of course the inability to differentiate definitively between pneumonia or influenza).
[6]
This led us to the following thoughts:
If we can’t leverage recent travel or test kits as key differentiators from Influenza cases, what data can we use to find unconfirmed COVID-19 cases? If we had additional data, what data modeling techniques would be leveraged for identifying cases to support understanding ofspread of the infection?
When considering hypothetical data sources, we wanted to lead with extracting symptomatic data from relevant patient interactions - such as clinician-staffed call center discussions or provider assessment documentation - and how analyzing these interactions increase the accuracy of projecting the spread of infection. Let’s walk through how this might look.
Generating a Hypothetical Dataset for Symptom-Diagnoses Pattern Analysis:
Following the uptake of clinical notes to a secured instance (we’ll save Teradata’s interoperability enablement for other context), we first connect
Teradata Vantage ML Engine to a known text analysis engine, for example here we show AWS Medical Comprehend, but you could also leverage the
Teradata MDM toolkit with our
NLP Toolkit for a custom approach. We then need to focus on generating a pattern that leverages common symptoms or patient presentations that infer a diagnosis.
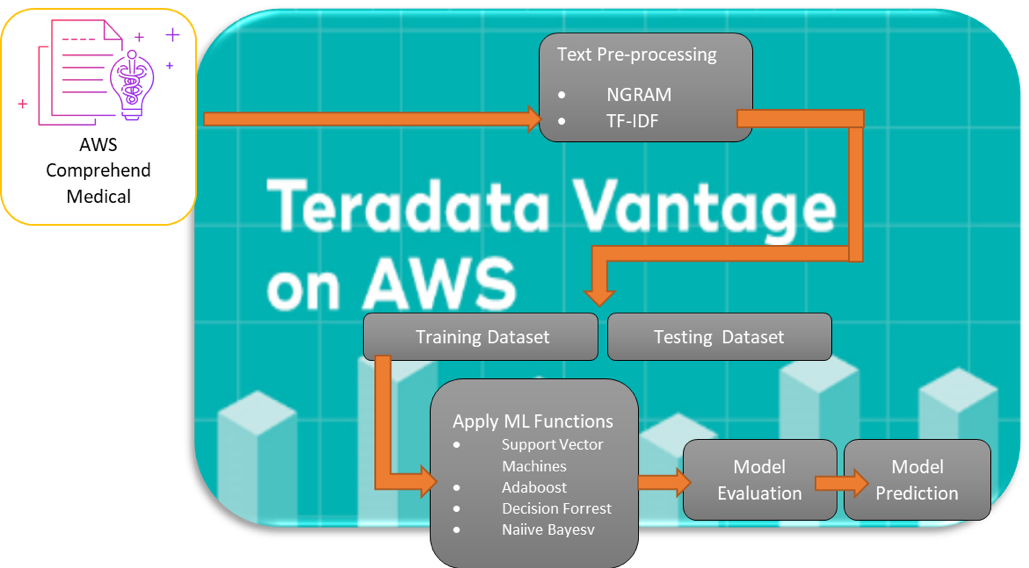
Once the text analysis engine receives the unstructured clinical narrative, it assigns medical ontology attributes to the note. In doing so, it produces structured clinical features that can file back to Teradata Vantage’s ML Engine package as an input to pre-processing and model development.
These inferred diagnosis are not coded by any provider by hand of course, and this process isn’t to be leveraged to diagnose any individuals. This process, sometimes referred to as ‘ghost coding,’ then feeds our training and testing datasets, where we’ll evaluate the modeling through confidence factors and standard metrics such as ROC curve.
Visual A: A provider assessment processed from Teradata to AWS Medical Comprehend that potentially indicates either seasonal Influenza or COVID-19 symptoms.
Pre-Processing and Predictive Classification Modeling Techniques for Text:
While healthcare organizations have generated sustainable data models focused classifying underdiagnosed Influenza cases with natural language processing (NLP)
[7], COVID-19 classifier data models are clearly new and still somewhat undependable. We’ll need to leverage what clinical assumptions we know regarding variations in symptomatic data between Influenza and COVID-19, in order to achieve a confidence level that differentiates COVID-19 cases from Influenza cases.
Visual B: Many individuals with COVID-19 present with similar set of symptoms to Influenza and Allergies.[8]
We use the TF-IDF scores derived from a training document set, in this case making assumptions based on the term frequency generated by healthcare agencies noted in Visual B, to create a model in a classification function (for example, SVMSparse (ML Engine)) and then use the resulting TF-IDF scores in a classification prediction function (for example, SVMSparsePredict_MLE).
Predictive algorithms used for health record text analysis are found in Vantage’s ML Engine
[9] and focus on establishing a scoring probability for each sample. In this scenario, if we establish two scoring probabilities, one for a COVID-19 class and another for an Influenza class, we can make certain assumptions as to whether the case is more likely one versus the other, or not at all. Whether choosing to use AdaBoost or SVM functions, the ability to fit and tune the training model against a number of algorithms at the same time to help speed up analysis.
Hypothetical Result Discussion:
With the text notes of both seasonal Influenza +/- diagnosis and COVID-19 and +/- negative diagnosis Teradata Vantage can take a set of symptoms and classify whether a case is either Influenza or COVID-19 with a percentage of probability. This process is an example of how public and government agencies can baseline their local or national epidemic spread to track pandemic potential of cases for which there are clinical interactions.
With a prediction like this we could potentially show the development of the ghost diagnosis based on extraction of symptoms that align with the patient episode. The total number of cases would be higher that confirmed by tests and may have lead decision makers down a different course.
Visual C: The simulation shows how the projected conversion of unconfirmed cases to confirmed cases provided by the model, versus the reported cases within the United States. The gray band represents the earliest known cases beginning earlier than the first reported case, representative of all epidemics lacking identifiable confirmation mechanisms.
Next: Impact on Bed Occupancy Rates
We mentioned a simulation that the Wuhan data, indicates a staggering 86% of cases in the population are undocumented, and therefore strain on US health systems are likely to occur faster and more frequently than anticipated.
[10] Many social barriers and resource indices will impact this public health risk. For the next post, we will get into the data and focus on the impact we are seeing on localized
inpatient bed occupancy rates across the country, and include analysis of
network chain of infections and
contact-tracing.
Jack McCush provides extensive analytic capabilities to a wide range of companies, including time series modeling, customer demand forecasting, and price elasticity. He has a broad background in customer behavior analysis, with expertise in developing customer value models that help build loyalty and retention by improving frequency and duration of customer visits. McCush has also provided Teradata customers with various services from Text Mining, Natural Language Process, Topic Modeling, as well as Naïve Bayes and Support Vector Machine classification models.
View all posts by Jack McCush
Mr. Ulatowski, an epidemiologist formerly of the Department of Health Science Research at Mayo Clinic, focuses on applying analytic techniques for a variety of client strategic initiatives, primarily centered on patient and member insight programs for healthcare and life science organizations. His accomplishments include leading the design of quality outcomes programs for two accountable care organizations, genetic engineering and research in therapy design for treatment of West Nile virus, and implementing a human factors engineering program assessing adoption of digital technology across health centers supporting underserved patients for a large west coast provider organization. His current initiatives focus on merging statistical analysis of both the social science and clinical data. Educated at the University of Wisconsin-Madison, his interests include understanding the impacts of human behavior on both epidemiologic studies and health policy.
View all posts by Dan Ulatowski