Did you know that the English language consists of over 200,000 words? Of these 200,000 words, many have the same meaning but different spelling (synonyms). Some words even have the same spelling but different meanings (homographs). Confusing, right?
Let’s imagine you were talking to your colleagues about your recent trek to the coast and you wanted to explain the faults you saw. Are you talking about errors in a system or geological faults? To further complicate your chat, what if you used ‘faults’ whilst your colleagues use ‘cracks’ to define the same geological phenomena? It’s a wonder we ever understand each other at all.
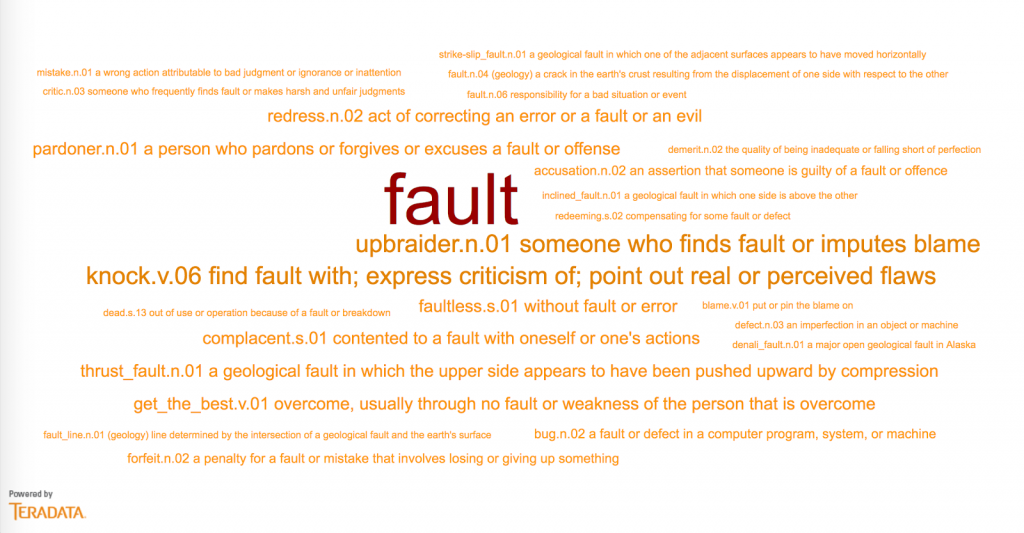
Figure 1: Fault, with all related synsets and definitions
Generally, we don’t confuse one another, because we’re aware of the different terms for things and often understand the context of both the situation being described and the conversation serving to describe it (context is a whole topic in itself that we’ll address another time).
But every term for a thing (a noun) actually sits within a hierarchical structure that connects every noun to every other noun, from the least generic nouns to the most generic, called an entity.
Of all things, 43.9 percent have multiple nouns to describe the same thing. Whilst 18.7 percent of nouns are homographs — spelt the same, but with differing definitions. Experience teaches us to identify these words and when to use the right one. To look at this structure in detail, you can clearly see just how complicated the network of nouns each of us navigates each day really is.
The hierarchy is made up of synonyms and hyponyms (think of it like a synonym that increases specificity). Direct synonyms are words that have the same meaning, such as fault and crack. Their synset, or sets of synonyms, is fault.n.04, where the first part is the word that relates them, the “n” states it is a noun, and 04 means it is the fourth definition of fault. In this example, the words are found on the same level in the hierarchical structure of nouns, however not all words on the same level are synonyms of each other.
Levels in the structure are based on the generality of the noun. Entity is the most general noun or root hypernym, which all nouns lead up to. If we traverse down the hierarchy, going down to each hyponym, we get to more specific words, such as San Andreas Fault and inclined fault.
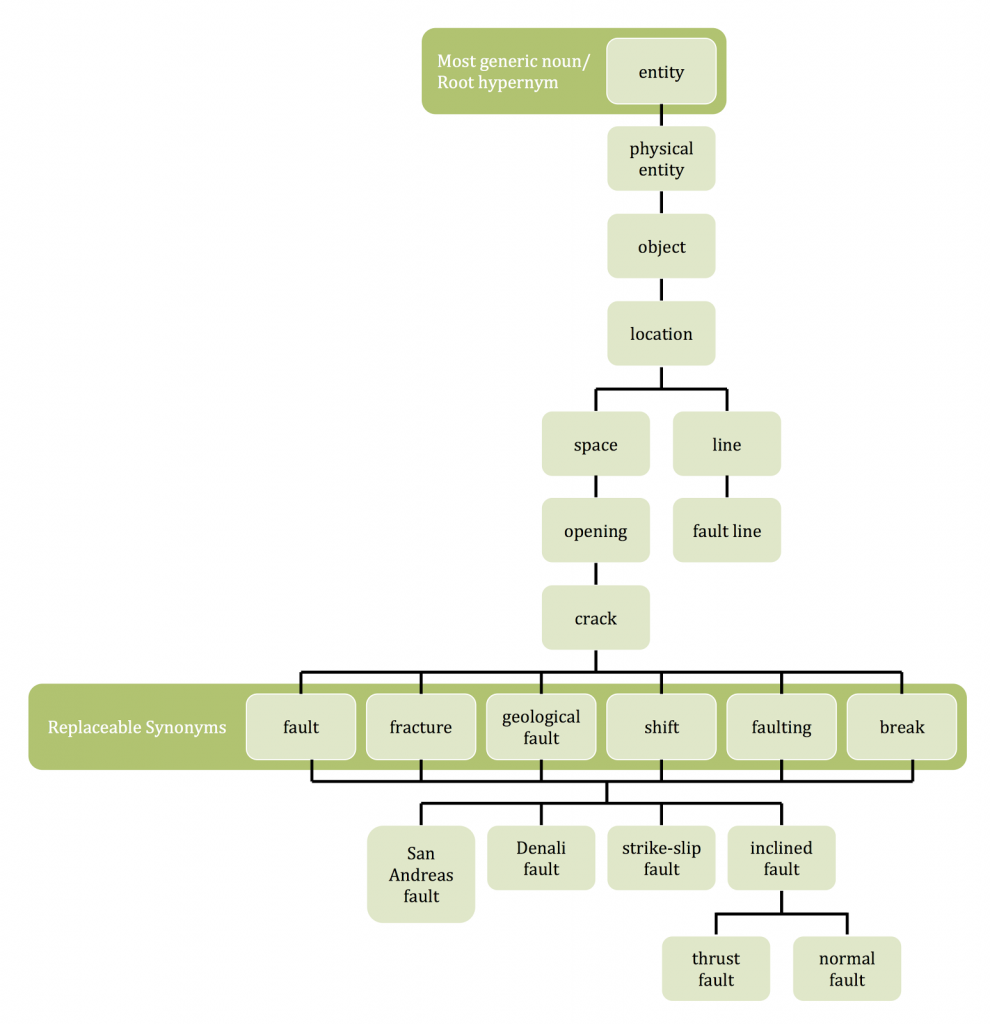
Figure 2: The hierarchy related to the synset fault.n.04
The English language is confusing enough for humans to learn and use to communicate with each other; experience teaches the meaning of words and when and where to use them. But how can a computer cope with this structure? How does a computer determine the intended definition of a word?
Today it is becoming commonplace for us to rely on computers to process text information. If a computer could specify word use using related hypernyms, it could differentiate between homographs, narrowing down exactly what is meant. Conversely, it could also work out when two words are actually referring to the same thing.
The English language may contain thousands of words with multiple definitions and spellings, but it is structured. This structure could hold the key to helping computers understand exactly what is being said. What could we do with this? Translate documents better? Identify new ways of matching documents for plagiarism? What if we could use the knowledge of words being used to determine sentiment (both positive and negative) and stop online bullying?
Take a look at language analytics in action with the Art of Analytics.
As a relatively new addition (since March 2017) to the Teradata team, Elise Hampton works as a data scientist within Think Big Analytics. At the Research School of Astronomy & Astrophysics in Australia, Elise first encountered machine learning, which has driven her to shift careers from astronomical research to data science. Elise’s interests (in data science) include finding ways of using machine learning to 'do the hard work for us’, using computers to understand language and people, and solving the world’s problems. Outside of work you may find Elise seeking out famous people at Sci-fi conventions, pondering whether lightsabers will ever be possible, and cosplaying as Disney Princesses or pop-culture heroes around Australia.
View all posts by Elise Hampton